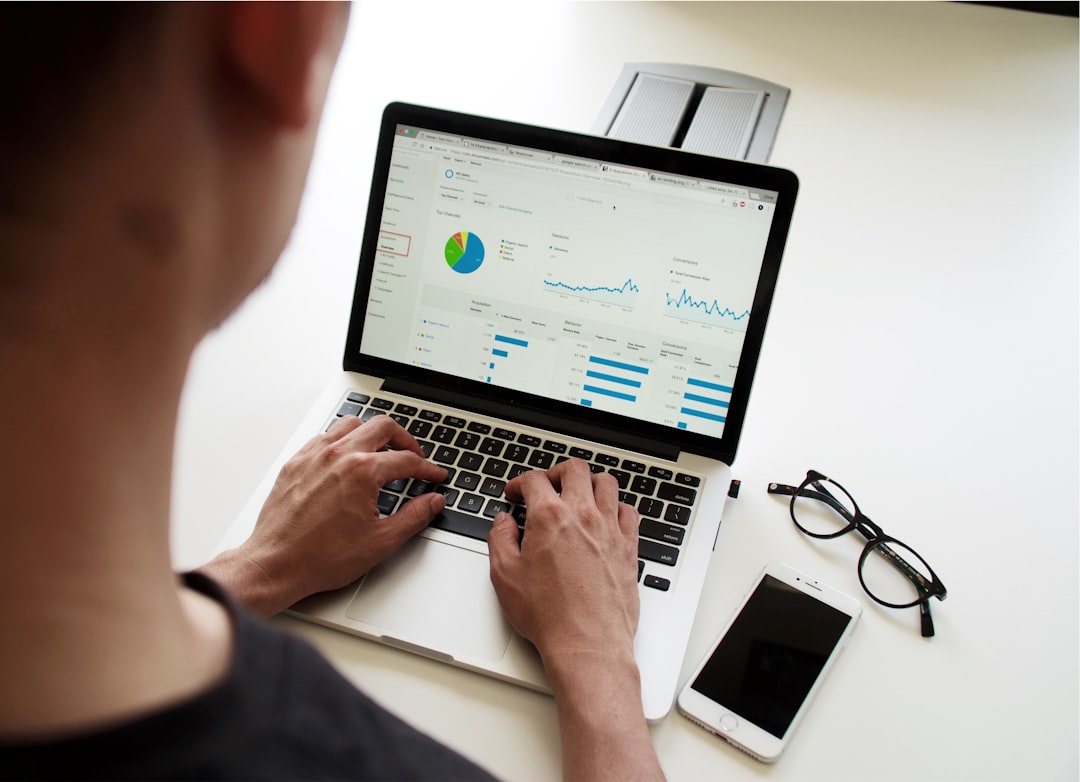
Dynamic Batch Processing for Centralized Data Management
In today’s data-driven world, organizations are inundated with vast amounts of information generated across various channels. Effective management of this data is crucial for businesses to make informed decisions and maintain a competitive edge. One of the most effective methodologies is Dynamic Batch Processing for Centralized Data Management. This approach allows organizations to process data in batches while adapting to changing workloads and business requirements.
Understanding Dynamic Batch Processing
Dynamic Batch Processing is a strategy that enables the collection, processing, and analysis of data in batches, rather than one piece at a time. This method allows for efficient handling of large volumes of data and minimizes the latency often experienced in real-time processing.
Benefits of Dynamic Batch Processing
- Efficiency: By processing data in batches, organizations can reduce the overhead associated with individual transactions, leading to improved performance.
- Scalability: As data volume increases, dynamic batching can adapt to the workload, making it easier to scale operations without significant infrastructure changes.
- Resource Management: Dynamic batch processing optimizes resource usage, ensuring that processing power is allocated based on demand.
- Centralized Data Management: This approach streamlines data from different sources into a centralized system, making it easier to access, analyze, and derive insights.
Key Components of Centralized Data Management
Centralized Data Management (CDM) serves as the backbone of dynamic batch processing. It involves consolidating data from multiple sources into a single repository. Key components include:
Data Integration
Integrating data from various sources—such as databases, cloud storage, and third-party APIs—ensures a cohesive dataset that can be easily processed and analyzed.
Data Quality
Ensuring data quality is paramount. Organizations must implement validation, cleansing, and transformation processes to maintain the integrity of their data before it enters the batch processing system.
Data Governance
Data governance frameworks help organizations define policies and procedures for data management, including security, compliance, and access controls.
Emerging Trends in Dynamic Batch Processing
As technology evolves, so do the methodologies surrounding data management. Here are some emerging trends that are shaping the future of dynamic batch processing:
Cloud-Based Solutions
With the rise of cloud computing, organizations are increasingly adopting cloud-based data management solutions. These platforms offer scalability, flexibility, and cost-effectiveness, making them ideal for dynamic batch processing.
Machine Learning and AI
Integrating machine learning and artificial intelligence into dynamic batch processing can enhance predictive analytics capabilities. By analyzing historical data trends, organizations can optimize batch sizes and processing schedules.
Real-time Analytics
While dynamic batch processing primarily focuses on batch processing, there is a growing demand for real-time analytics. Combining both methodologies allows organizations to gain immediate insights while still benefiting from batch efficiency.
Practical Applications of Dynamic Batch Processing
Dynamic Batch Processing finds its application across various industries:
E-commerce
E-commerce platforms utilize dynamic batch processing to analyze customer behavior, manage inventory, and optimize pricing strategies based on recent purchasing trends.
Healthcare
In the healthcare sector, dynamic batch processing can be employed to streamline patient data management, ensuring that healthcare providers have access to the most up-to-date information for decision-making.
Financial Services
Financial institutions leverage dynamic batch processing for transaction monitoring and fraud detection, allowing them to process and analyze large volumes of financial data quickly.
Case Study: Netflix
Netflix’s recommendation system is a prime example of dynamic batch processing in action. By analyzing user viewing patterns in batches, Netflix can deliver personalized content recommendations, enhancing user engagement and satisfaction.
Tools and Resources for Dynamic Batch Processing
To implement dynamic batch processing effectively, organizations can leverage various tools and technologies such as:
- Apache Spark: A unified analytics engine that facilitates large-scale data processing.
- Apache Kafka: A distributed streaming platform that can handle real-time data feeds, integrating them into batch processing workflows.
- Amazon Redshift: A cloud-based data warehouse that allows for efficient batch processing and analytics.
For further exploration, consider reading:
Conclusion
Dynamic Batch Processing for Centralized Data Management is an essential strategy for organizations looking to harness the power of their data efficiently. By adopting this approach, businesses can improve their data management processes, gain valuable insights, and ultimately drive better decision-making.
Embrace the potential of dynamic batch processing in your organization today. Consider trying out the tools mentioned to enhance your data management capabilities and stay ahead in the competitive landscape.
Glossary of Terms
- Dynamic Batch Processing: Processing data in adaptable batches based on workload requirements.
- Centralized Data Management: Managing data from multiple sources in a single repository.
- Data Integration: Combining data from different sources into a unified format.
- Data Governance: Frameworks and policies for managing data quality, security, and compliance.
By leveraging the principles of dynamic batch processing, organizations can navigate the complexities of data management and unlock new opportunities for growth and innovation.